TensorFlow Privacy : Learning with Differential Privacy for Training Data
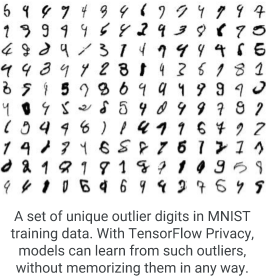
Introducing TensorFlow Privacy: Learning with different privacy for training data Today, we are excited to announce TensorFlow Privacy (GitHub), an open-source library that makes it easier for developers to not only train machine-learning models with privacy but also to advance the state of the art with machine learning. Strict privacy guarantee. Modern machine learning is increasingly used to create amazing new technologies and user experiences, many of which involve training machines to learn responsibility from sensitive data, such as personal photos or emails. Ideally, the parameters of trained machine-learning models should encode general patterns rather than facts about specific training examples. To ensure this, and to give strict privacy guarantees if the training data is sensitive, it is possible to use technology based on different privacy principles. In particular, when trained in user data, those technologies offer strict mathematical guarantees that the model wil...